I recently attended the Major Projects Association event: “The Unresolved Issue of Estimating and Forecasting”, (which was a great event) where I asked a question to the panel “whether Cost Managers were the right people to produce cost forecasts at the early stage of infrastructure projects, or whether instead, we should utilise a more data-scientific approach instead”.
I won't provide the panel response to my question as I am keen to hear your thoughts - please feel free to leave your answer in the comments.
However, what I will do is share some of my thoughts that led me to ask the question in the first place.
Early project forecasts
The typical approach used to produce a forecast at the earliest project stage (Strategic Outline Case etc) is a “base cost + uplift” approach. The base cost is usually produced using a “should cost model” approach (in my experience, a bottom-up parametric type model), and then an uplift (often Optimism bias) is applied to mitigate the challenges associated with low project definition and high uncertainty associated with early project forecasts (see fig 1).
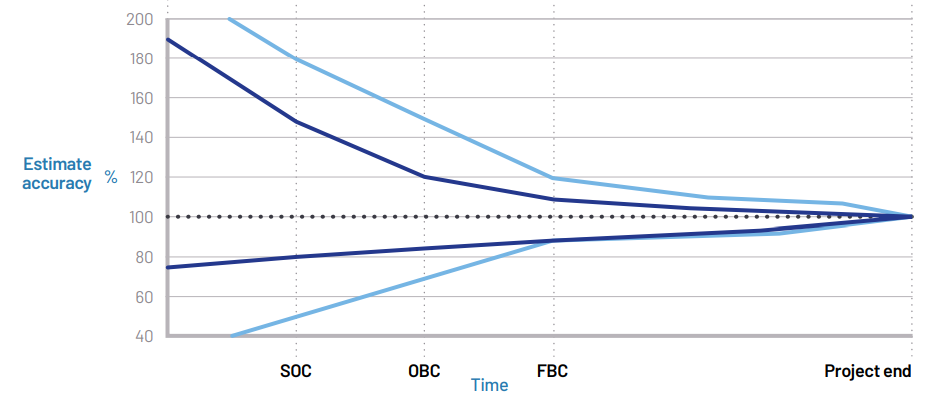
Fig 1. Diagram showing the typical accuracy of estimates over a project lifecycle
In my opinion, there are several issues around Optimism Bias as per HMT Greenbook. Firstly, as stated in a Greenbook, Optimism Bias it is not a financial contingency tool/application (although often regarded as one) and secondly, it is a blunt tool, (generic values) and may not be representative of your project – given the limited options available.
Whereas, Reference Class Forecasting (RCF) offers a robust statistical and project-oriented methodology for calculating uplifts, employing a systematic top-down approach rooted in historical data and outcomes from analogous, previously executed projects and in my mind, should be employed where possible over HMT Optimism Bias factors, which HMT also recommends this (please feel free to reach out on all things RCF).
“5.45 Ideally adjustments should be based on an organisation’s own evidence base for historic levels of optimism bias. In the absence of robust organisation-specific estimates generic values are provided” HMT Greenbook
Another key factor that needs consideration when producing an early project stage forecast, is the availability and even accessibility of project-specific data (ie ground conditions, benchmark data etc) to those producing cost forecasts.
The absence of data (ie scope) presents an opportunity for those producing cost models to be influenced by their heuristics and cognitive biases, potentially undermining their capacity to produce accurate and robust forecasts. However, some may argue that a key benefit of cost professional expertise is that they can “fill in the blanks” by providing their own insight based on their experience. I do think that there is merit to the argument and therefore value in the expertise, but we also have to remember:
I think the issues raised in the paragraph above are further exacerbated for linear infrastructure ie transport routes, new transmission networks, etc over a single site/location asset ie a prison/hospital etc
My hypothesis, feel free to challenge, is that when you start to add scale both in terms of size, number of assets, multiple sites, and multiple complexities and trying to capture these elements in multiple cost models, then there is a potential for “information overload” that may “shutdowns the brain” when trying to figure out the complex independencies, the various characteristics and traits, increase level of optimism bias and then we do what we humans do best – we take mental shortcuts (heuristics) and try to bring order to the scale and complexity – and perhaps this can lead to mistakes such as the underestimation of projects.
Whereas, Reference Class Forecasting (RCF) offers a robust statistical and project-oriented methodology for calculating uplifts, employing a systematic top-down approach rooted in historical data and outcomes from analogous, previously executed projects and in my mind, should be employed where possible over HMT Optimism Bias factors, which HMT also recommends this (please feel free to reach out on all things RCF).
“5.45 Ideally adjustments should be based on an organisation’s own evidence base for historic levels of optimism bias. In the absence of robust organisation-specific estimates generic values are provided” HMT Greenbook
Another key factor that needs consideration when producing an early project stage forecast, is the availability and even accessibility of project-specific data (ie ground conditions, benchmark data etc) to those producing cost forecasts.
The absence of data (ie scope) presents an opportunity for those producing cost models to be influenced by their heuristics and cognitive biases, potentially undermining their capacity to produce accurate and robust forecasts. However, some may argue that a key benefit of cost professional expertise is that they can “fill in the blanks” by providing their own insight based on their experience. I do think that there is merit to the argument and therefore value in the expertise, but we also have to remember:
- project maturity is low,
- we are humans, and we can be overconfident in our abilities and
- perhaps it is not their place or role to fill in the gaps (potentially baking in new/not-needed assumptions into the project)
I think the issues raised in the paragraph above are further exacerbated for linear infrastructure ie transport routes, new transmission networks, etc over a single site/location asset ie a prison/hospital etc
My hypothesis, feel free to challenge, is that when you start to add scale both in terms of size, number of assets, multiple sites, and multiple complexities and trying to capture these elements in multiple cost models, then there is a potential for “information overload” that may “shutdowns the brain” when trying to figure out the complex independencies, the various characteristics and traits, increase level of optimism bias and then we do what we humans do best – we take mental shortcuts (heuristics) and try to bring order to the scale and complexity – and perhaps this can lead to mistakes such as the underestimation of projects.
Data-led approach for early project forecasting
The “base cost + uplift model”, mentioned above is one approach. Another, as recommended by the Infrastructure Projects Authority, is the use of top-down benchmarks using data from previously completed projects, for early project-stage forecasting. This data will include any cost escalation incurred in the project, therefore providing a “de-bias” and more representative forecast and starting position. Importantly, this approach can provide decision-makers with robust (top-down) models that can be spun up quickly to cover an array of project and scope options i.e. what the cost of an additional 50km of track, or 4 prisons instead of 3 to support and underpin robust and multiple option testing for the economic case (option appraisals), helping to reduce/mitigate the "linear project overload" issue (as impacts of independencies have been realised in the data).
The diagram below (fig 2) sets out the level of effort/input between data scientists and cost professionals at the project stage. Both roles should be utilised throughout the project lifecycle, but the effort of resources may depend on the project lifecycle/maturity. This approach also may provide tension and validation of the models between data-scientists and cost professionals, both bringing their divers expertise and insight into the knotty problem of robust early cost forecast.
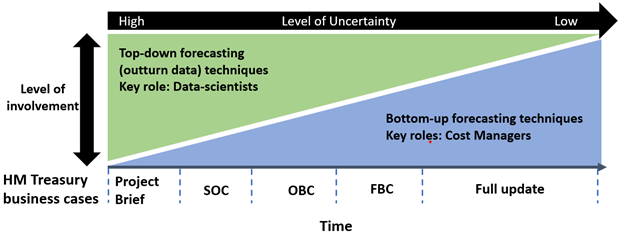
Fig 2. Model depicting the effort of roles for producing forecasts across UK Government Project lifecycle
But why data scientists?
My reflection is that data scientists are better equipped to produce data-driven forecasts and equipped to navigate issues around variability, and bias. They are able to define the appropriate metrics based on the approach, and importantly the validity of the modelling and forecasting methods.
Why this is important? The levels of uncertainty, presence of noise, and underlying biases within the data are significant at early project stages and this requires careful consideration and deliberation over the approach and the tools used (given the project maturity and understanding at the early stage - ie Montecarlo may not be appropriate).
Data Scientists are not only able to identify these potential obstacles in forecasting but they can look into quantifying what is unknown by providing confidence levels in regard to predictions and furthering insights on the deliverability and performance of the project and its range of likely outcomes (leveraging top-down and completed project data).
Lastly, through “regression analysis” you can quantify the relationships between identified cost drivers and project costs by calculating coefficients. If you have data on potential cost drivers ie access constraints, project location (brownfield vs. greenfield), site security, procurement methodology, regional influences, and project complexity rating etc you can investigate their impact on the overall project cost. Allowing you to test “Does it make the boat go faster”, or “Does it make the project cheaper”
The resulting coefficients indicate the extent of cost variation when comparing two scenarios: one with the inclusion of a specific cost driver and one identical in all aspects except for the exclusion of that driver. A positive coefficient signifies that utilising the cost driver leads to cost escalation, while a negative coefficient implies that the cost driver results in cost reduction.
I am not advocating the move away from cost professionals at the early project stage and replacing them with data scientists (a lot of my friends are cost managers). My article title talks about an “evolution” and not a revolution. I think there is huge value in having both professions working openly and collaboratively for the benefit of the project and project team (right tools for the right job – at the right stage) and in the future, perhaps with changes in training and curriculum, we may see a convergence of these skills and expertise in a new role Cost-data-engineer, Cost-data-scientists? You read it here first…!
Lastly, I hope that the above article sets out the value and benefits of data for organisations and their projects. In my next blog, I will set out some of the challenges, considerations, and opportunities for collecting data.
In the meantime, if you would like to know more or would like a discussion on the above, please feel free to contact me aleister.hellier@oxfordglobalprojects.com. Follow me for more exciting blogs and the second part of this blog (I know you can't wait!)
Stay in the loop
Sign up to hear about our new courses
Thank you!